Reviewed
publications
Spinner, S., Rudnik, R., Herr, M., Probst, A., Radespiel, R.: Scale Resolving Simulation of Buffet Effects Induced by Ultrahigh Bypass Ratio Nacelle Installation. Journal of Aircraft, published online February 04, 2025
https://arc.aiaa.org/doi/epdf/10.2514/1.C038119
CEAS Special Issue zum FOR 2895 Symposium
CEAS Aeronautical Journal, Volume 15, Issue 1, January 2024
Special Issue: Numerical and experimental studies on high speed stall phenomena
Issue Editors: Thorsten Lutz, Andrea Beck, Lars Koop
https://link.springer.com/journal/13272/volumes-and-issues/15-1?utm_source=toc&utm_medium=email&utm_campaign=toc_
13272_15_1&utm_content=etoc_springer_20240323
Lürkens, T., Meinke, M., Schröder, W.: Impact of 2D engine nacelle flow on buffet. CEAS Aeronaut J 15, 23–35 (2024). https://doi.org/10.1007/s13272-024-00728-8
Herr, M., Radespiel, R., & Probst, A.: (2023) Improved Delayed Detached Eddy Simulation with Reynolds-stress background modelling. Computers & Fluids, 265 (106014). Elsevier. doi: 10.1016/j.compfluid.2023.106014. ISSN 0045-7930.
Herr, M., Probst, A. & Radespiel, R.: (2023) Grey area in embedded wall-modelled LES on a transonic nacelle-aircraft configuration. CEAS Aeronautical Journal, Seiten 1-18. Springer. doi: 10.1007/s13272-023-00664-z. ISSN 1869-5590.
A. Waldmann, M. Ehrle, J. Kleinert, D. Yorita and T. Lutz: "Mach and Reynolds number effects on transonic buffet on the XRF-1 transport aircraft wing at flight Reynolds number", Exp Fluids 64, 102, 2023.
doi: 10.1007/s00348-023-03642-7
Blind, M., Kleinert, J., Lutz, T., & Beck, A. (2023). A Time-Accurate Inflow Coupling for Zonal LES. Accepted by CEAS Aeronautical Journal. https://doi.org/https://doi.org/10.48550/arXiv.2301.03192
Kleinert, J., Ehrle, M., Waldmann, A. Lutz, T.: “Wake Tail Plane Interactions for a Tandem Wing Configuration in High-Speed Stall Conditions”, submitted to CEAS Aeronaut J, https://doi.org/10.48550/arXiv.2301.05760, preprint, 2023.
Herr, M., Probst, A., & Radespiel, R.: Grey area in Embedded WMLES on a transonic nacelle-aircraft configuration, submitted to CEAS Aeronautical Journal, Preprint, https://doi.org/10.48550/arXiv.2301.05299
Herr, M., Radespiel, R., & Probst, A. (2023). Improved Delayed Detached Eddy Simulation with Reynolds-Stress Background Modeling, submitted to Computers & Fluids, Preprint https://doi.org/10.48550/arXiv.2301.07223
Christopher Julian Schauerte and Anne-Marie Schreyer: Experimental analysis of transonic buffet conditions on a two-dimensional supercritical airfoil, AIAA Journal, under revision (2023)
Christopher Julian Schauerte and Anne-Marie Schreyer: Experimental investigation on the turbulent wake flow in fully-established transonic buffet conditions, submitted to CEAS Aeronaut J, Published 02 November 2023; DOI: https://doi.org/10.1007/s13272-023-00690-x
Zahn, R., Weiner, A. and Breitsamter, C.: Prediction of Wing Buffet Pressure Loads Using a Convolutional and Recurrent Neural Network Framework, accepted for publication in CEAS Aeronautical Journal, CANJ-D-22-00126, 2022.
Blind, M., Kopper, P., Kempf, D., Kurz, M., Schwarz, A., Beck, A., & Munz, C.-D. (2022). Performance Improvements for Large Scale Simulations Using the Discontinuous Galerkin Framework FLEXI. Accepted by High Performance Computing in Science and Engineering'22. Springer.
Blind, M., Kahraman, A. B., Larsson, J., & Beck, A. (2022). Residual Estimation for Grid Modification in Wall-Modeled Large Eddy Simulation Using Unstructured High-Order Methods. Submitted to Computers & Fluids. https://doi.org/https://doi.org/10.48550/arXiv.2301.03199
Kleinert, J., Stober, J., Lutz, T.: “Numerical Simulation of Wake Interactions on a Tandem Wing Configuration in High-Speed Stall Conditions”, CEAS Aeronaut J Dez. 2022, https://doi.org/10.1007/s13272-022-00634-x
Spinner, S., Rudnik, R., “Experimental Assessment of Wing Lower Side Buffet Effects Induced by the Installation of a UHBR Nacelle”, CEAS Aeronautical J. (2022), https://doi.org/10.1007/s13272-022-00632-z.
Zahn, R., and Breitsamter, C.: Airfoil Buffet Aerodynamics at Plunge and Pitch Excitation Based on Long Short-Term Memory Neural Network Prediction, CEAS Aeronautical Journal, Vol. 13, pp. 45-55, 2022; https://doi.org/10.1007/s13272-021-00550-6.
Zahn, R., and Breitsamter, C.: Prediction of Transonic Wing Buffet Pressure Based on Deep Learning, CEAS Aeronautical Journal, 2022; https://doi.org/10.1007/s13272-022-00619-w.
Lutz, T. Kleinert, J., Waldmann, A., Koop, L., Yorita, D., Dietz, G.: “Research Initiative for Numerical and Experimental Studies on High Speed Stall of Civil Aircraft“, Journal of Aircraft, Nov. 2022, https://doi.org/10.2514/1.C036829
Herr, M., & Probst, A. (2021). Efficient Modelling of Near-Wall Turbulence in Hybrid RANS-LES Simulations. In Notes on Numerical Fluid Mechanics and Multidisciplinary Design (Vol. 151, pp. 615–624). https://doi.org/10.1007/978-3-030-79561-0_58
Rozov, V., and Breitsamter, C.: Data-Driven Prediction of Unsteady Pressure Distributions Based on Deep Learning, Journal of Fluids and Structures, Vol. 104, 2021; https://doi.org/10.1016/j.jfluidstructs.2021.103316
Zahn, R., Linke, T., and Breitsamter, C.: Neural Network Modeling of Transonic Buffet on the NASA Common Research Model, NNFM XIII, Springer Verlag, Vol. 151, pp. 697-706, 2021.
Andre Weiner and Richard Semaan: flowTorch - a Python library for analysis and reduced-order modeling of fluid flows, Journal of Open Source Software, 6(68), 3860, 2021, https://doi.org/10.21105/joss.03860
Conference
papers
Lürkens, T., Meinke M., Schröder, W.: Analysis of Upstream Traveling Waves on Transonic Buffet. DLES14 - Direct and Large Eddy Simulation, 2024
Herr, M., Probst, A., Spinner, S., Rudnik, R. & Radespiel, R.: (2023) Scale Resolving Simulation of Nacelle / lower-Wing Interferences near High Speed Stall Conditions. Dt. Luft- und Raumfahrt Kongress DLRK 2023, 2023-09-19 - 2023-09-21, Stuttgart.
Lürkens, T., Meinke, M., Schröder, W.: Wall-Modeled LES of Buffet under the Influence of Engine Nacelle Flow. Deutsche Gesellschaft für Luft- und Raumfahrt - Lilienthal-Oberth e.V., DLRK, 2022, https://doi.org/10.25967/570433
Spinner, S., Rudnik R., „Vortex Interaction in Transonic Flow for Wing-Mounted UHBR Nacelles”, 2023, AIAA SciTech Forum, 23–27 January 2023, National Harbor, MD, AIAA Paper to be published.
Herr, M., Spinner, S., Probst, A., Rudnik R., & Radespiel R., Embedded WMLES of transonic buffet on a nacelle-aircraft configuration AIAA SciTech Forum, 23–27 January 2023, National Harbor, MD, AIAA Paper 2023-0246, https://doi.org/10.2514/6.2023-0246, 2023.
Christopher Julian Schauerte and Anne-Marie Schreyer: Influence of Reynolds Number on Transonic Buffet Conditions on a Supercritical Airfoil, AIAA 2023-0431, AIAA SCITECH 2023 Forum, January 2023, https://doi.org/10.2514/6.2023-0431
Zahn, R., Weiner, A. and Breitsamter, C.: Wing Buffet Pressure Load Prediction Based on a Hybrid Deep Learning Model, 33rd Congress of the International Council of the Aeronautical Sciences (ICAS), Paper ICAS2022-0043, Stockholm, Sweden, 2022 (Paper & Presentation awarded with McCarthy Student Award).
Christopher Julian Schauerte, Johannes Bosbach, Robert Konrath, Reinhard Geisler, Florian Philipp, Anne-Marie Schreyer: Characterization of Transonic Buffet on a Swept Wing by Means of Cryogenic PIV, Deutsche Gesellschaft Für Luft- Und Raumfahrt - Lilienthal-Oberth E.V., DLRK, September 2022, https://doi.org/10.25967/570369
Christopher Julian Schauerte and Anne-Marie Schreyer: Characterization of shock buffet on a supercritical 2D airfoil in transonic flow, 20th International Symposium on Application of Laser and Imaging Techniques to Fluid Mechanics, Lisbon, July 2022.
Zahn, R., and Breitsamter, C.: Prediction of Transonic Wing Buffet Pressure Based on Deep Learning, 70. Deutscher Luft- und Raumfahrtkongress (DLRK), Paper 0033, Bremen, 2021.
Spinner, S., Rudnik. R., “Design of a UHBR Through Flow Nacelle for High Speed Stall Wind Tunnel Investigations”, Deutscher Luft- und Raumfahrt Kongress 2021, Deutsche Gesellschaft für Luft- und Raumfahrt-Lilienthal-Oberth e.V., https://doi.org/10.25967/550043. urn:nbn:de:101:1-2021100112224829096665 (2021).
Herr, M., Radespiel, R., & Probst, A. (2021). Erweiterung einer skalenauflösenden RSM-IDDES Methodik zur Erforschung von Verkehrsflugzeugkonfigurationen. In 20. STAB-Workshop-Jahresbericht 2021 (pp. 79-80).
Zahn, R., and Breitsamter, C.: High-Speed Buffet Aerodynamics Modeling Based on a Long Short-Term Memory Neural Network, 69. Deutscher Luft- und Raumfahrtkongress (DLRK), Paper 0027, 2020.
Daniel Fernex, Andre Weiner, Bernd Noack and Richard Semaan: Sparse Spatial Sampling: A mesh sampling algorithm for efficient processing of big simulation data, AIAA Best Paper Award, AIAA 2021-1484, AIAA Scitech 2021 Forum, January 2021, https://doi.org/10.2514/6.2021-1484
Andre Weiner and Richard Semaan: Simulation and modal analysis of transonic shock buffets on a NACA-0012 airfoil, AIAA 2022-2591, AIAA SCITECH 2022 Forum, January 2022, https://doi.org/10.2514/6.2022-2591
Data analysis
tool flowTorch
flowTorch - a Python library for analysis and reduced-order modeling of fluid flows
The flowTorch library [1, 2] enables researchers to access, analyze, and model fluid flow data from experiments or numerical simulations. Instead of a black-box end-to-end solution, flowTorch provides modular components allowing to assemble transparent, automated, and reproducible processing workflows with ease. Fluid flow data coming from experiments (PIV, iPSP, Schlieren) or simulations (TAU, Flexi, OpenFOAM) may be accessed via a common interface in a few lines of Python code. Internally, the data are organized as PyTorch tensors. Relying on PyTorch tensors as primary data structure enables fast array operations, parallel processing on CPU and GPU, and exploration of novel deep learning-based analysis and modeling approaches. The flowTorch documentation includes a rich collection of Jupyter notebooks [3] demonstrating how to apply the library components in a variety of different use cases, e.g., finding coherent flow structures with modal analysis or creating reduced-order models.
POD and DMD
POD and DMD modes being characteristic for shock-boundary layer interactions. The dominant Buffet frequency is around 340Hz.
Comparative view of experimental surface pressure and DMD reconstruction.
The image shows the dominant pattern (mode) in the unsteady surface pressure distribution on the XRF1 model. Both proper orthogonal decomposition (POD) and dynamic mode decomposition (DMD) are available in flowTorch for the analysis of unsteady flows.
The DMD decomposes the flow into several characteristic spatial flow structures, so-called modes. Each mode is associated with a unique frequency. By superposition of a few dominant modes close to the buffet frequency, the main flow features can be recovered, as seen in the animation.
[1] https://doi.org/10.21105/joss.03860
[2] https://github.com/FlowModelingControl/flowtorch
[3] https://flowmodelingcontrol.github.io/flowtorch-docs/1.0/index.html
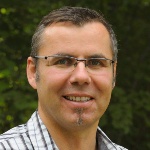
Thorsten Lutz
Dr.-Ing.Head of working group Aircraft Aerodynamics / Head of working group Wind Energy